How to Measure AI Impact in Workplace Safety: Metrics That Matter in 2025
- Shoyab Ali
- 11 hours ago
- 7 min read
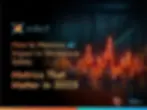
In 2024, a construction site in Hong Kong installed AI cameras to catch PPE violations. Within the first 30 days, over 500 unsafe acts were flagged, and the management celebrated what they thought was a breakthrough in workplace safety.
Fast forward three months:
Violations doubled.
Accident frequency remained the same.
Worker morale? Crashing.
The reality?
They weren’t solving problems—they were just counting them.
In 2025, industries like construction, mining, oil & gas, and manufacturing have one question driving their safety agendas:
Is detecting and reporting safety breaches enough, or are we merely documenting risk instead of eliminating it?
Why AI-Powered “Detection” Isn’t Delivering the Impact You Think
AI is fantastic at identifying when a worker steps into a restricted zone or forgets a hard hat. But here’s what you don’t see in the dynamic dashboards:
Bogus Data: A Fortune 500 manufacturer found 23% of its safety alerts were false positives due to outdated data feeds. AI was reporting issues that no longer existed.
Complexity Overload: Safety teams are often seen to struggle to translate AI outputs into actionable steps due to the vast load of information and it’s not so common inferences. If your AI needs its own “translator,” it’s not helping.
Alert Fatigue: At a Singaporean construction site, AI flagged an average of 200 violations/day. Within weeks, supervisors stopped reviewing them altogether—critical warnings drowned in noise.
Siloed Information: PPE violations tracked, but no connection to shift schedules, environmental data, or permit-to-work (PTW) statuses. Single-source data leads to single-point failures.
As a result, high-risk companies collect a mountain of data, plenty of reports, and zero reduction in serious injuries and fatalities (SIFs).
But as we navigate through the complexities of deploying AI in workplace safety, there is a better way to track the safety breaches and turn them into important pieces of information that are holistic and strategically sound.
Metrics That Actually Matter in 2025 (and Why They’re Game-Changers)
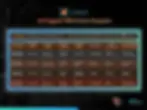
To move beyond numbers and drive impact, AI safety management systems must align with standardized safety metrics—but not just report them—predict and prevent.
Let’s break down the metrics redefining safety strategies this year:
1. Total Recordable Incident Rate (TRIR)
What it measures:
The number of OSHA-recordable incidents per 200,000 working hours.
What’s changed with advanced AI-powered safety solutions:
Predictive Modelling uses AI cross-references like productivity heatmaps, seamless approval logs, IoT data (worker heart rates, temperature, gas leak chances, chemical levels, etc.), and historical trends to predict potential spikes in incidents.
Instead of reactive post-incident analysis, using comprehensive data from Digital Works Supervision System (DWSS) help AI predict TRIR spikes 48–72 hours in advance.
2. Lost Time Injuries (LTI)
What it measures:
The number of injuries that result in time away from work due to severity.
The new age AI Boost:
The integration with behaviour based safety systems helps to identify unsafe worker behaviour at any time. Furthermore, the future of safety in modern workplaces is being shaped by advanced devices like smart watches that monitor worker vitals in real time, adding critical data points for proactive health and safety management.
Vision AI integration allows ergonomic assessments when the worker is in their tasks. It analyzes unsafe movements, such as improper lifting techniques, repetitive motions or unsafe posture, and correlates them with shift schedules.
When worker fatigue patterns from past and present data emerge, AI triggers schedule modifications to prevent injury before it occurs.
Interesting Case Example: A global mining company reported a 45% drop in fatigue-related LTIs after AI systems flagged at-risk workers and prompted rest cycle adjustments.
3. Days Away, Restricted, or Transferred (DART)
What it measures:
The number of cases where employees are unable to perform their full duties due to injury, which can include restrictions (e.g., lighter duties) or transfers to different roles following an injury.
What’s different now:
Predictive AI combines data from AI video analytics, IoT sensors, incident management software, and real-time environmental monitoring. Through them, AI can detect early signs of conditions that may lead to a DART-level incident.
For example, AI can identify unsafe behaviors such as workers jumping and running around or showing signs of mishandling machinery.
Proactive Alerts help detect trends such as overexposure to hazardous conditions or frequent tasks with a risk of musculoskeletal diseases that increase the likelihood of an injury or illness.
Advanced Analytics with AI integrates with machine learning algorithms that analyze data from past incidents, worker health records, and external environmental factors to predict risks across shifts or teams, allowing safety managers to intervene before injuries occur.
4. Serious Injuries and Fatalities (SIF)
What it measures:
The number of high-severity incidents or fatalities on the job.
AI’s Role:
Pattern Recognition using AI analyzes near-misses, ensures auto compliance monitoring, and unsafe worker-machine interactions to identify patterns of behavior and unsafe conditions that lead to SIFs.
Using this data, AI constantly generate LLM-powered predictive insights, identifying critical moments of tasks such as in high-rise work schedules before they escalate into severe incidents.
5. Injury Frequency Rate (IFR) and Injury Severity Rate (ISR)
What it measures:
How often injuries occur per million hours worked, and the average severity of injuries in the workplace.
AI-Driven Value:
Identifying Unsafe Trends based on data across shifts, job roles, and operational zones. This helps in risk profiling, which is based on every worker individually, instead of an overview report.
This proactive approach enables safety managers to shift focus based on the severity levels to specific teams or areas showing signs of increased risk.
Predictive Escalation with AI uses this data to predict which minor injuries are most likely to escalate into more severe or long-term injuries, prompting early intervention.
The AI-Powered Unified Safety Scorecard: From Metrics to Meaning

Imagine an EHS dashboard that converts 1000s of data points into a single, actionable safety score — real-time, easy to read, and predictive. Each of the safety metrics can be converted into simple insights through a centralized management platform.
Here is how it looks:
Metric | Weight (%) | Score Range (0-100) | Input | AI Normalized Score |
TRIR (Total Recordable Incident Rate) | 25% | Lower TRIR = Higher Score | 2.1 vs OSHA Avg. 3.0 | 85 |
LTI (Lost Time Injuries) | 15% | Fewer LTIs = Higher Score | 1 case this month | 80 |
SIFs (Serious Injury & Fatalities) | 20% | Zero SIFs = 100 | 2 flagged incidents | 60 |
DART (Days Away, Restricted, or Transferred) | 15% | Lower DART = Higher Score | 1.5 rate | 75 |
IFR (Incident Frequency Rate) | 15% | Lower = Better | 1.8 vs benchmark 2.5 | 78 |
ISR (Incident Severity Rate) | 10% | Lower = Better | 4.2 severity index | 65 |
Final Safety Score | 75.9 |
As the final safety score is calculated from the weighted average of the individual metrics, the AI-powered safety surveillance dashboard is quick enough to analyse the total safety score and suggest corresponding strategic recommendation as below.
Safety Score | Risk Level | Metric | AI Insight | Quick Strategy |
75.9 | 🟡 Moderate | TRIR LTI SIFs DART IFR ISR | Toolbox talk + supervisor check-ins PPE alert system + smart signage Re-audit + scenario-based drills Digital PTW reminders via wearables Retraining + shift rotation Emergency drills + gamified alerts |
This new AI metric for workplace safety excels because:
Combines all data, not just visual or numeric
Real-time and predictive — lets EHS managers act before incidents
Personalized —workers using conversational AI get their risk feedback with micro-training recommendations on-site
So, Is Detecting Safety Breaches Enough?
Maybe 2 years ago.
But in 2025, when AI can predict near-misses, highlight invisible risks, and drive interventions before something happens, simply detecting is no longer sufficient.
viAct’s AI-powered safety ecosystem is redefining how risk is managed in heavy industries with a Unified Safety Scorecard — turning individual safety metrics into one clear, predictive score for faster, smarter decisions.
Metrics are no longer just backwards-looking indicators of past incidents — with the integration of AI layers such as Computer Vision, IoT, LLMs, Edge AI and Generative AI, they transform into forward-operating controls.
A unified safety intelligence system enables the cross-referencing of previously siloed data sources, from permit logs and environmental sensors to worker behavior analytics and incident histories, generating real-time situational awareness.
This convergence empowers safety teams with predictive insights, where AI not only flags emerging risks but also recommends targeted mitigation actions, enabling a more proactive, data-driven approach to safety governance across heavy industries.
The real question is: Are you ready to shift from reaction to prediction?
Quick FAQs
1. How can AI improve the accuracy of safety metrics like TRIR and LTI in heavy industries?
AI in workplace safety can transform traditional safety metrics like TRIR and LTI by providing predictive insights. By integrating data from sources like PTW systems, IoT sensors, and AI-driven wearables, companies can identify patterns of unsafe behavior before incidents happen, reducing recordable injuries and lost time injuries.
2. What role does AI play in predicting and preventing Serious Injuries and Fatalities (SIFs) on construction sites?
An AI-powered safety management system uses real-time data from computer vision and sensor networks to monitor high-risk areas and worker behavior. By analyzing near-misses and unsafe interactions, AI can predict potential SIFs, allowing EHS teams to take proactive steps and significantly reduce the likelihood of catastrophic incidents.
3. How can integrating AI with DART and TRIR data enhance proactive safety measures in high-risk environments?
Integrating AI with metrics like DART (Days Away, Restricted, or Transferred) and TRIR provides a holistic view of safety performance. AI can cross-reference historical data, worker fatigue, and environmental risks to identify high-risk conditions, enabling more targeted interventions and reducing both injury severity and downtime.
4. In what ways can AI prevent workplace incidents related to fatigue, leading to a reduction in LTIs and DART rates?
AI systems, such as wearable devices and computer vision, can monitor fatigue levels and unsafe movements in real-time. By adjusting work schedules and alerting managers to potential fatigue-related risks, AI helps prevent LTIs and DART incidents, ensuring better worker safety and productivity.
5. How does a Unified Safety Scorecard powered by AI enhance overall workplace safety management?
A Unified Safety Scorecard integrates multiple safety metrics like TRIR, LTI, SIFs, ISR, and DART into a single real-time dashboard. By cross-referencing data from IoT sensors, AI, and historical logs, it provides a comprehensive view of site safety, allowing teams to track risks, make informed decisions, and implement proactive measures to reduce incidents across the board.
Ready to Turn Safety Metrics into Strategic Moves?
Read More: